Improving data centre operations with AI and Machine Learning
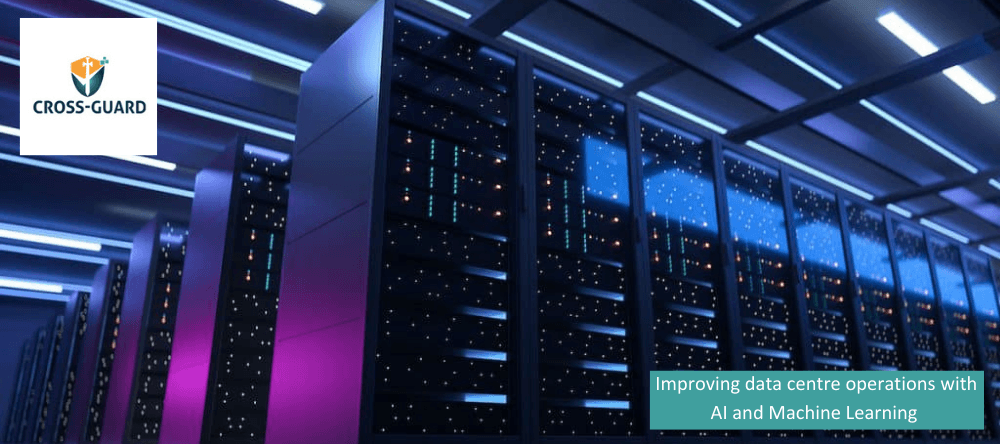
Data centres are under pressure. Growth of the Internet of Things, the rise of remote working, 5G rollouts, the demand for high-performance streaming services — these are just a few of the ways our digital lives are putting data centres under increasing strain to meet growing data storage demands. It’s a problem that AI and machine learning are tackling head on.
One problem after another
The problem of rapidly growing workload volumes placed on data centres is exacerbated by the fact that of the roughly 8000 data centres worldwide, a significant number are a decade old or more. This is because the average lifespan of a data centre is 20 years. And this means that many data centres operating today were not built with the current data volume challenges in mind. Nor were they designed to accommodate changes in data centre technology, such as the latest cooling systems and new approaches for sustainability.
The challenges today are therefore, two-fold: data centres need to operate more efficiently to meet increasing data service demands and they need to operate in a more environmentally friendly and energy efficient way to tackle the issues of climate change.
Falling short of requirements
The current approach to meet these requirements is falling short. To meet data service demands, energy efficiency is often sacrificed. Results from the UK’s largest data centre thermal optimisation survey reveal that over three-quarters of UK data centres aren’t compliant with ASHRAE’s thermal guidelines and around one in every 10 IT racks in the data centres surveyed were operating outside of ASHRAE’s recommended temperature range. Research suggests this isn’t due to a lack of cooling capacity. It’s caused by poor monitoring that leads to inefficient cooling.
Unfortunately, traditional data centre monitoring simply isn’t up to the task. Sensors provide information on conditions at their specific location but conditions can vary between sensors, sometimes significantly. Traditional monitoring, therefore, provides trend data but not granular details. This isn’t enough to regulate energy efficiency in a meaningful way; a way that allows specific actions to be taken to solve problems. This is where AI and machine learning can make all the difference.
AI and machine learning solutions
Unlike traditional monitoring, machine learning can gather data at a granular level and when combined with 3D visualisations that highlight changes can provide meaningful, real-time data on performance.
When it comes to action, combining the granular data provided by machine learning with AI analytics can provide insights that support real-time energy efficiency decisions.
These insights aren’t just about immediate adjustments, such as changing fan speeds and set point temperatures, but also larger changes for long-term efficiency, such as changing floor grille layouts and rack locations.
This isn’t just a theory. It’s already been put into practice with impressive results. Google’s DeepMind AI reduced their data centre cooling costs by 40% and reduced their energy consumption by 15%. Verizon is in on it too, as well as Equinix and other leading data centre providers. As the digital world grows, placing more pressure on data centres, AI and machine learning are catching up to relieve that pressure.
SHARE
DOWNLOAD A COPY OF OUR BROCHURE
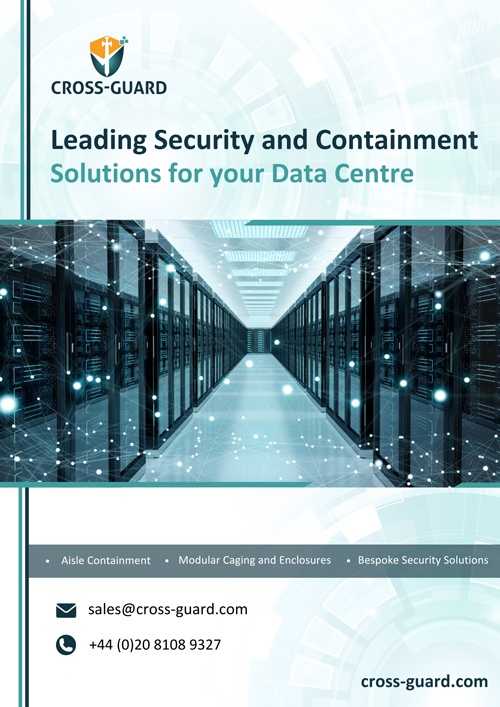